A few months ago, I remember reading some press about a new economics preprint out of MIT. The Wall Street Journal covered the research a few days after it dropped online, with the favorable headline, “Will AI Help or Hurt Workers? One 26-Year-Old Found an Unexpected Answer.” The photo for the article shows the promising young author, Aidan Toner-Rodgers, standing next to two titans of economics research, Daron Acemoglu (2024 Nobel laureate in economics) and David Autor.
“It’s fantastic,” said Acemoglu.
“I was floored,” said Autor.
The Atlantic and Nature covered the research as well, with both publications seemingly stunned by the quality of the work. And indeed, the quality of the work was stunningly high! The article analyzes data from a randomized trial of over one thousand materials researchers at the R&D lab of a US-based firm who were given access to AI tools. Toner-Rodgers adeptly tracks the effect of access to these AI tools on:
Not only do each of these metrics show really clear effects, but Toner-Rodgers throws every tool in the book at exploring them, using a number of really sophisticated methodologies that must have taken tremendous effort and care:
At the time I saw the press coverage, I didn’t bother to click on the actual preprint and read the work. The results seemed unsurprising: when researchers were given access to AI tools, they became more productive. That sounds reasonable and expected.
Toner-Rodgers submitted his paper to The Quarterly Journal of Economics, the top econ journal in the world. His website said that he had received a “revise and resubmit” already, meaning that the article was probably well on its way to being published.
MIT put out a press release this morning stating that they had conducted an internal, confidential review and that they have “no confidence in the veracity of the research contained in the paper.” The WSJ has covered this development as well. The econ department at MIT sent out an internal email so direly-worded on the matter that on first glance, students reading the email had assumed someone had died.
In retrospect, there had been omens and portents. I wish I had read the article at the time of publication, because I suspect my BS detector would have risen to an 11 out of 10 if I’d given it a close read. It really is the perfect subject for this blog: a fraudulent preprint called “Artificial Intelligence, Scientific Discovery, and Product Innovation,” with a focus on materials science research.
Hindsight is of course 20/20, but the first red flag that should have been raised is the source of the data itself. The article gives enough details to raise some intense curiosity. It’s a US-based firm that has (at least) 1,018 researchers devoted to materials discovery alone, an enormous amount. This narrows it down to a handful of firms. Initially the companies Apple, Intel, and 3M came to mind, but then I noticed this breakdown of the materials specialization of the researchers in the study:
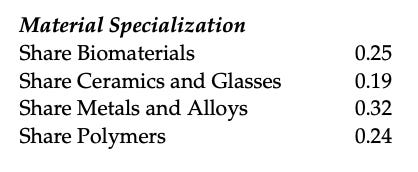
This was bizarre to me, as very few companies do massive amounts of materials research and which also is split fairly evenly across the spectrum of materials, in disparate domains such as biomaterials and metal alloys. I did some “deep research” to confirm this hypothesis (thank you ChatGPT and Gemini) and I believe that there are a few companies that could plausibly meet this description: 3M, Dupont, Dow, and Corning. None of these are perfect fits, either, especially with the 32% share on metals and alloys.
I’ll really be embarrassing myself if it turns out that an actual R&D lab was supplying Toner-Rodgers with data and he was just fraudulently manipulating it, but I think this is quite unlikely, and it’s more plausible that the data was entirely fabricated to begin with. I have several reasons for believing this:
The next red flag should have been how spotless the findings were. In every domain that was explored, there was a fairly unambiguous result. New materials? Up by 44% (p<0.000). New patents? Up by 39% (p<0.000). New prototypes? Up by 17% (p<0.001).
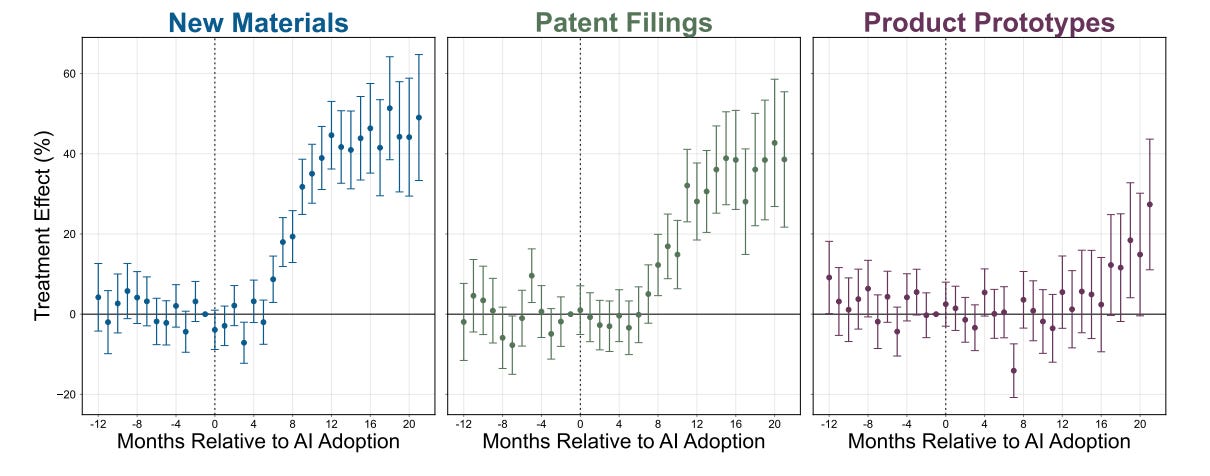
The quality of the new materials? Up, and statistically significant. The novelty of the new materials? Up, and statistically significant. Did researchers who were previously more talented improve more from AI tool use? Yes. Were these results reflected in researchers self-assessments of their time allocation? Unambiguously yes. The plot for that last bit is every economist’s dream, a perfect encapsulation of the principle of comparative advantage taking effect:
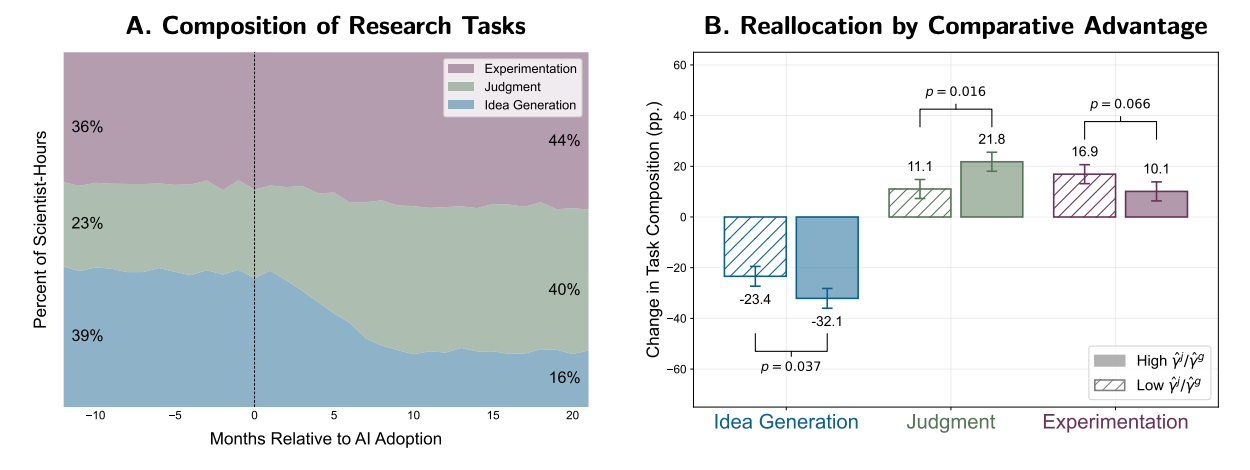
And look how contrived and neat this other plot looks, showing whether researchers’ self-assessment of their judgment ability correlates with their survey response on the role of different domains of knowledge in AI materials discovery. Three out of four categories show a neat increase and one out of four remains constant (which is the one that from first principles seems like it wouldn’t matter, experience using other AI-evaluation tools).
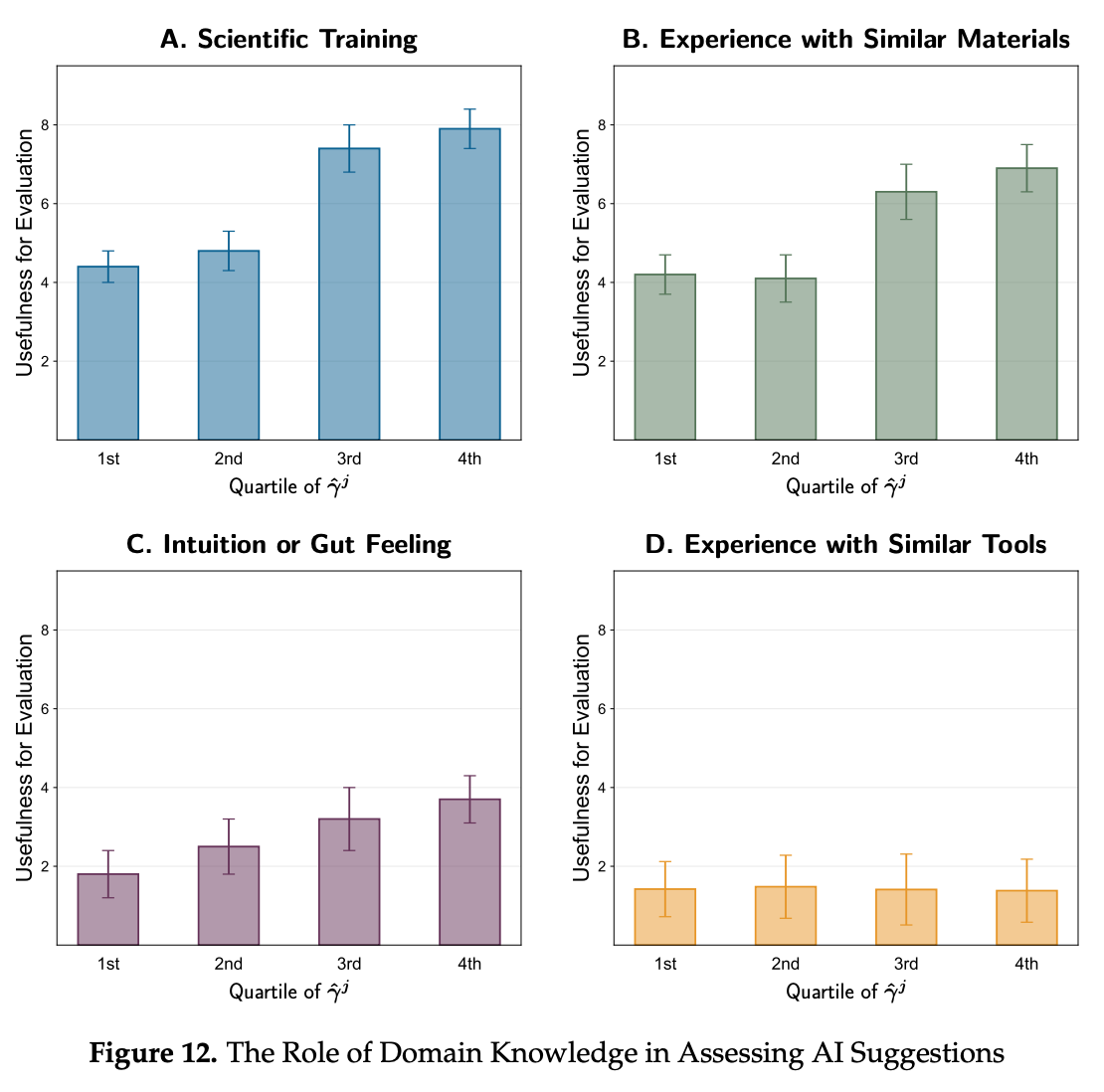
This plot also makes no sense, when you think about it. Why would researchers with better judgment be systematically more likely to give higher numbers on this survey question on average?
Q3: On a scale of 1–10, how useful are each of the following in evaluating AI-suggested candidate materials (scientific training, experience with similar materials, intuition or gut feeling, and experience with similar tools)?
And then, to cap it off, here’s how Toner-Rodgers describes a fortuitous round of layoffs at the firm, that miraculously doesn’t interfere with the data collection for the primary analysis and yet contributes an insightful example that supports his findings:
“In the final month of my sample—excluded from the primary analysis—the firm restructured its research teams. The lab fired 3% of its researchers. At the same time, it more than offset these departures through increased hiring, expanding its workforce on net. While I do not observe the abilities of the new hires, those dismissed were significantly more likely to have weak judgment. Figure 13 shows the percent fired or reassigned by quartile of γˆ j. Scientists in the top three quartiles faced less than a 2% chance of being let go, while those in the bottom quartile had nearly a 10% chance.”
I mean, come on, be for real…
Now, my background in materials science provides me a neat leg up, as I’d assume the vast majority of those reviewing/reading/following this paper are economists and people interested in the effects of AI use.
How do the parts of this paper that directly engage with materials science hold up? Well, they’re a little too clever. Take Toner-Rodgers’ analysis of “materials similarity” where he claimed to have used crystal structure calculations to determine how similar the new materials were to previously discovered materials. The plot is stunningly unambiguous, the new materials discovered with AI are more novel.
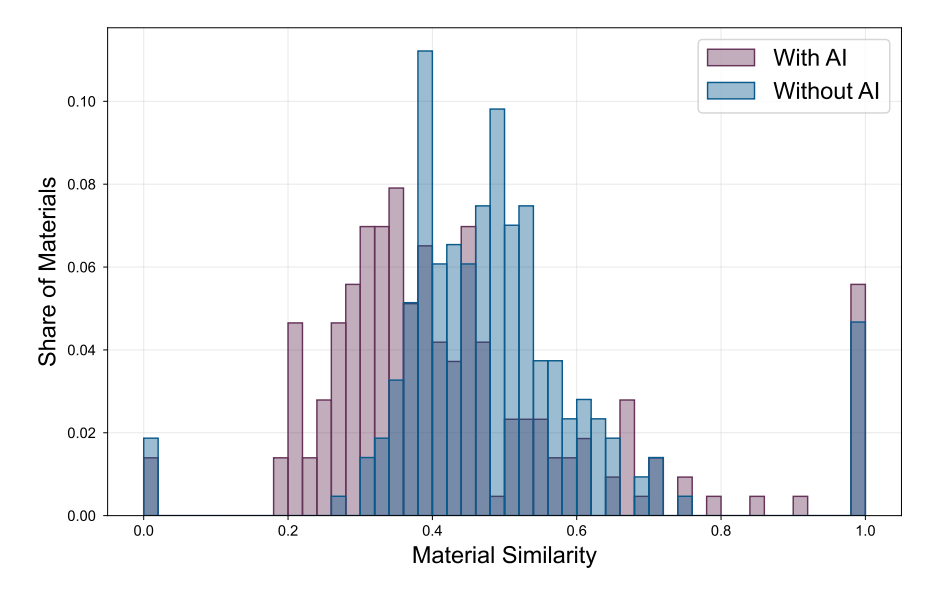
However, it boggles the mind that a random economics student at MIT would be able to easily (and without providing any further details), perform the highly sophisticated technique from the paper he cites (De et al, 2016), especially in this elegantly formalized manner without any domain expertise in computational materials research. This graph, and the data it represents, if true, would probably be worth a Nature paper on AI materials discovery on its own. In his paper, it’s relegated to the appendices.
This methodology also makes no sense at generalizing across different types of materials, so I have no clue how you could reduce the results from such broad classes of materials to a single figure of merit in this manner. The gaps between 0.0 and 0.2 and 0.8 and 1.0 might seem reasonable to someone who read a few papers and noticed similar gaps in a couple of the graphs, but it would be bizarre when generalized across several classes of materials, and the data is likely completely fabricated for this reason. To simplify this critique, a novel metal alloy would have a very different level of similarity from a reference class of previously-discovered alloys, than a novel polymer would from its own reference class. It would require some really sophisticated methodology to normalize this single figure of merit across material types, which Toner-Rodgers does not mention at all. Also, this would all be insanely challenging to implement using data from the Materials Project, requiring some sophisticated “big data” workflows. If you want a smoking gun, here’s a graph from a paper, Krieger et al, “Missing Novelty in Drug Development,” which Toner-Rodgers cites, using a similar methodology for drug discovery. It looks eerily similar to the distribution in this preprint. This distribution might make sense for drugs, but makes very little intuitive sense for a broad range of materials, with the figure of merit derived directly from the atomic positions in the crystal structure. This is the kind of mistake that someone with no domain expertise in materials science might make.
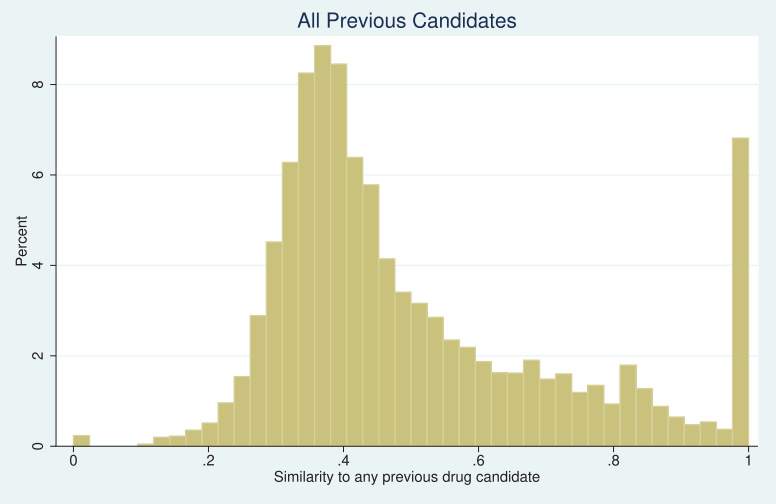
Toner-Rodgers’ treatment of “materials quality” would also probably drive a materials scientist insane if they were forced to think about it at length.
Here’s the equation he uses to calculate the “quality” of a new material:
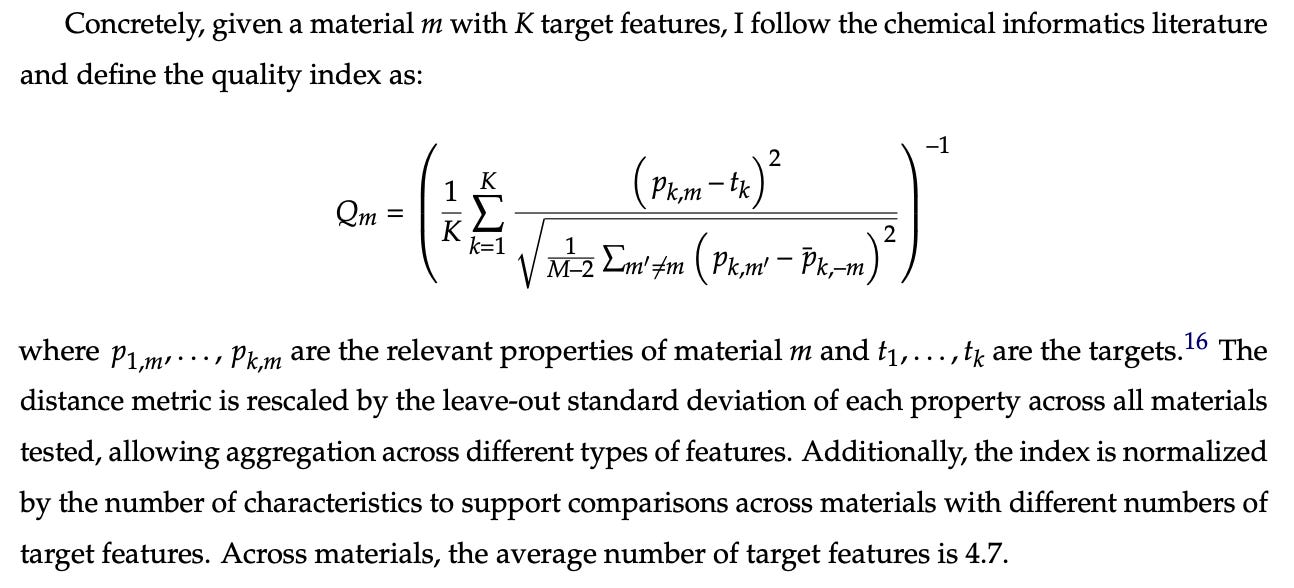
This would likely be a case of extreme garbage in: garbage out. First of all, there are typically no “target features” that are easily reduced to single values, but also, even if there were, some of these would be distributed on a log scale, which would dramatically skew the values for certain classes of materials. Also, in general, the “quality” of a new material that an R&D lab develops is likely not at all related to improvements in the actual top-line figures of merit like “band gap” or “refractive index”, the two examples that Toner-Rodgers gives. Instead, they would be for things like durability, affordability, ease of manufacture, etc. These are all properties that are not easily reduced to a single value. And even if they were, good luck getting researchers to measure, systematize, and document these values for the new materials!
However, from this amalgam of gibberish, Toner-Rodgers manages to extract a significant finding anyway! All 1,018 scientists contribute to this endeavor, and statistically significant findings are reported in every single category:
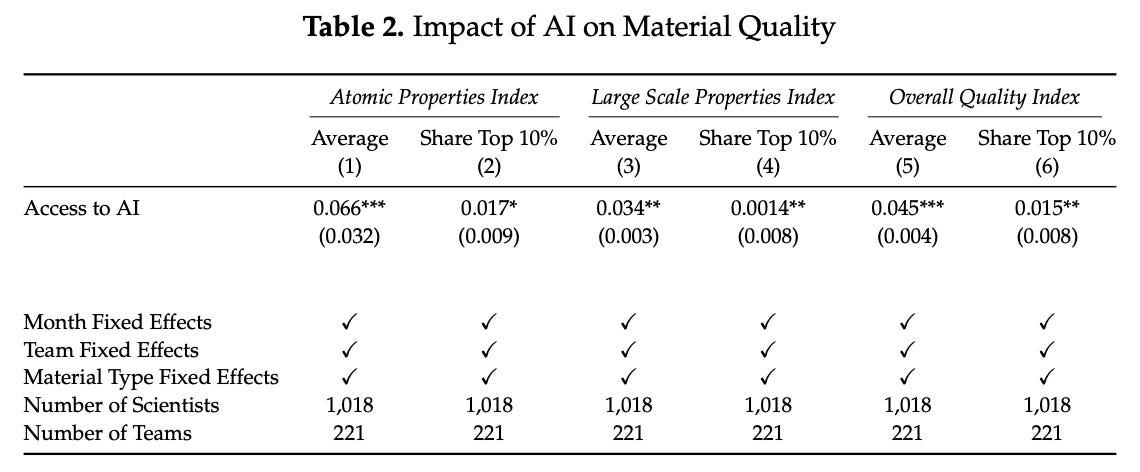
Some people might look at this saga and think “ah, another bs preprint, thankfully we have peer review to deal with it.” However, I think that were it not for the fact that this preprint had gained so much attention, this article would have slipped through peer review, only to embarrass the editors of the top econ journal in the world after being published and reported on.
Moreover, these are the kind of errors that the editorial process at an econ journal might not catch. I think the most clearly fraudulent components of the paper are those that seem to dramatically simplify the complexity of the materials work going into the paper. Robert Palgrave, who has been an outstanding critic in the past of skeptical work on AI materials discovery, has a twitter thread noting similar problems with the work (I promise I read his thread after writing the bulk of this blog post). And when the piece originally came out, he had an orthogonal, but also very valid set of reasons for being skeptical of the work (mostly due to the difficulty in defining the “novelty” of materials).
In general, the lesson I think we should learn is to be much more skeptical of these sorts of research findings. Learning new things about the world is hard, and generally randomized trials on such a complex topic should show much more ambiguous results. The fact that the data was so beautiful and fit such a perfect narrative should have raised alarm bells, rather than catapulting the results to international attention.
I also think that if , this could have led to a much more rapid conclusion to the fraud. Probably a materials scientist who read the paper realized this was fraudulent but wasn’t able to get that view quickly to the economists who were actually reading and discussing the paper. A well-written arxiv comment explaining why the data on materials similarity, for example, couldn’t be true, would have gone a long way.
After writing a draft of this blog post, I saw this tweet which says that Corning, this January, filed an IP complaint with the WIPO against Toner-Rodgers for registering a domain name called “corningresearch.com”.
This validates my earlier guess as to which companies’ data this might plausibly be. However, it looks like Toner-Rodgers may have been using this website to privately substantiate his fake data, without the knowledge of Corning? I’m not sure what this means, but it’s certainly interesting. It’s possible he was using the domain name to send fake emails to himself, or to generate pdf files at plausible-sounding urls, to show his advisor. Corning is a great company, and if they actually did collect this data and evaluate the materials properties in some coherent manner, that’s extremely impressive. However, I still think it’s far more likely that the data was completely fabricated by Toner-Rodgers.